The world we know today has come a long way in the last decade. There has been a multitude of fundamental differences in the way organizations function.The manner in whichtech giants like Google and Facebook have risen to the top of the stock exchange with the minimal sales of physical products is a telling sign of where the world is headed. A few years ago, current Google CEO SundarPichaidecided that it would be a good idea to incorporate data feedback loops into all products in the Google ecosystem. This was done at a time when data science had not yet grown into the behemoth that is has become today. This was back when it was still predominantly an academic disciple as it had been for decades. The simple idea behind this was that the most important resource for a tech giant like Google is the data it collects from its users. Its users span the entire globe – that is a lot of data that has to be managed globally – not an easy task even ten years ago. The transformation that Google has been able to achieve on the back of that decision has been phenomenal, and data lies at the heart of that transformation. How was it that billion and billions of rows of simple data was able to bring about this metamorphosisof a search engine into a complete ecosystem of products which are now ubiquitous?We know it wasn’t by threatening its users or blackmailing them. Then how? There are of course a lot of reasons, butMachine learning and Artificial intelligence were pivotal.
What is Machine learning? What is Artificial intelligence? To answer both these questions, we have to go a long way back. Back to when business intelligence was ruling the roost when it came to data driven decision making in organizations. Organizations get an understanding of their performance and areas of improvement by ‘reporting the past’ – generating reports on a regular basis about the significant KPI’s of organizational functions. Business Intelligence is still a practice that continues to be used in almost all organizations and is not going to die anytime soon, as it does serve a very important purpose –a posteriori analysis. What has changed, or rather what is new is the scale and speed at which data is now being captured, thanks to new technologies for capturing and using these data sources. What has also changed is how we now use this data to ‘predict the future’.Unless you have been living under a rock these past few years, none of this is probablyvery new to you. Oversimplified, this is called the realm of machine learning . This is where models and algorithms are used to predict future occurrences based on the data that we have now, with a model being trained to fit and generalize based on the training data. There are multiple disciplines within machine learning, with varied real word applications such as speech recognition, computer vision, robotics, scientific research, pricing models, sales forecasts, and a range of other business problems.
What about that other buzzword we’ve been hearing a lot lately – data science? How is that different from machine learning?In layman’s terms, data or decision science is the set of practices that are followed to make decisions out of the predictions that machine learning has provided. It includes machine learning and everything around it that makes machine learning useful. This can be described as ‘creating the future’. In this case it would mean that for a given business problem, the relevant data is collected from varied sources, cleaned, labelled, used to build predictive models, and the predictions are then used to make real decisions. Data science has machine learning as an integral part and uses the predictive models to make useful decisions. Predictive modelling is only one part of data science though, as it includes other assignments such as finding hidden patterns in data. Data science is an amalgamation of multiple disciplines such as statistics, mathematics, computer science and information theory. These days, we often hear the phrase, ‘data is the new oil’. Some prefer to use the phrase, ‘LABELLED Data is the new oil’. The phrase does have a significant element of truth, though. Finding labelled data for accurate modelling is one of the most challenging aspects in this area. There are even organizationsthat employ people tasked solely with labelling data, making it usable for running reliable models.
Now let’s see where Artificial intelligencefits into this already crowded picture. Following the analogies that we have used till now, with business intelligence being‘reporting the past’, machine learningbeing‘predicting the future’, and data science being‘creating the future’, we can describeartificial intelligence as ‘exploring the future’. With AI, we should be able to computationally evaluate the outcomes of multiple scenarios and choose the best course of action based on the success measures that are set for the activity. The field of AI was born 50 years ago. Today we are in the second phase of AI which is supported by big data and cloud computing. Today AI can do things that we could not even have imagined 20 years ago! It can play chess and we have found that no human on the planet can play better than a machine. No human on the planet can play ‘Jeopardy’ better than a machine and recently an AI program even beat the best human ‘Go’ player. But is AI only good for beating humans at our own games? Can it do something more useful? These games are the field in which new AI algorithms are created and perfected. But using these algorithms we can do a lot more than feel bad about our own intellect. For example, within a few years, it is almost inevitable that we will see self-driving cars on our roads anddrones delivering packets for e-commerce services. Forget the scientist and the intellectual, AI is also on track to put artist to shame. AI has recently been getting into ‘creative’pursuits long though of as beyond the capabilities of machines. However, some recentpaintings created by deep networks have been terrific! AI is taking over our world (literally, according to some people’s fears) like never before.
Let us take a step back and decide a very relevant questionregarding . “When can a computer be called Intelligent?” Alan Turing, widely regarded as the father of theoretical computer science and artificial intelligence, was perhaps the first to address this problem comprehensively. He came up with an experiment known as the ‘Turing test’, which was based on the idea that a computer can be considered to be intelligent if it can‘dupe’ a human into believing that it is human. If we go by that definition, a recently created bot named Google Duplex has shown the ability to call restaurants and engage in conversations with a believably human-like voice to make table reservations. This can be considered as a good example of an AI application to have passed the Turing test, and it certainly put a lot of people at unease. It is also to be noted that we have been seeing a recent surge in the number of startups in the field of AI. The “.ai” domain name is one of the fastest selling domain names at present, indicating the widespread interest that AI has generated due to the recent advances in computational processing and GPU programming. Some of these startups do not actually have any AI functionality in any of their products, but they still want to take advantage of the buzz.
An important distinction between Data Science and AI is that, while data science deals with specific problem statements such as “What is causing the customer to churn?” and “How can driving behavior be optimized to maximize fuel efficiency?”, AI deals with scenarios which are much more general and grander in scale, such asself-driving cars and intelligent chatbots. Even as we make vast advances in the fields of machine learning and artificial intelligence, there is still a long way to go before a real ‘General Purpose AI’ is created – which could possibly be as conscious as a human. Such a machine would be described as possessing Artificial General Intelligence(AGI), where the machine is intelligent enough to perform any mental task that a human can. The pace of technological advancement is accelerating exponentially, and the times ahead hold much promise regarding humans addressing age-old problems with the aid of AI. It is only fair to say that AI is here to stay, whether you like it or not.
ML and AI
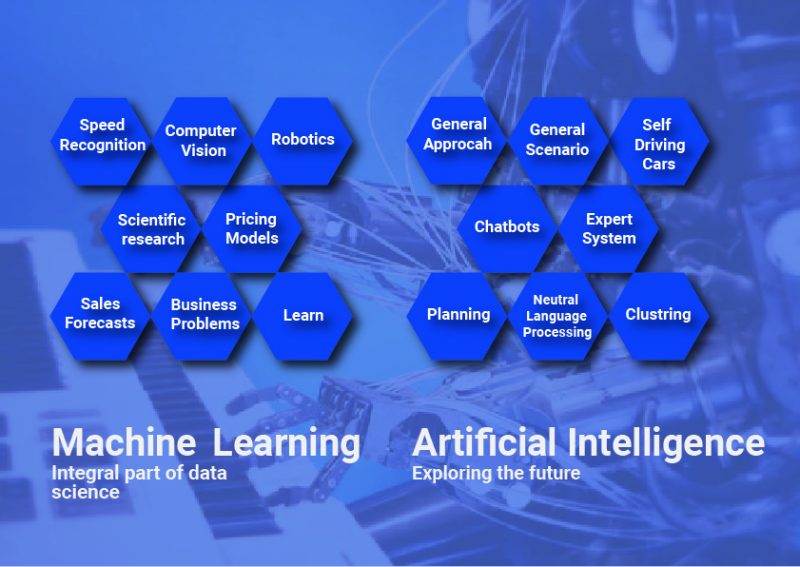